Examining the Engineers' Toolbox: AI Utilisation through Low-Code
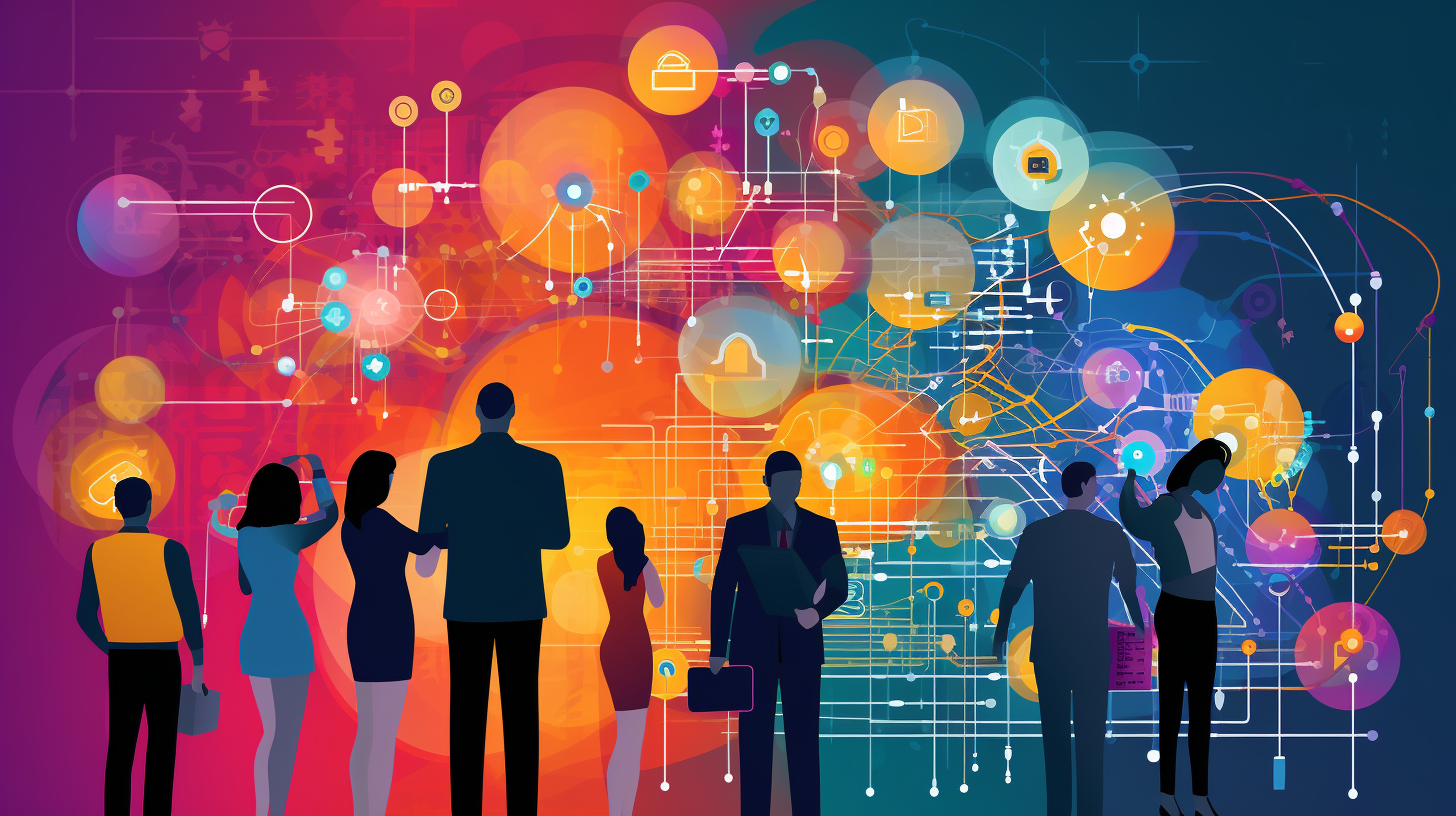
With the ongoing advancements in artificial intelligence (AI) and its widespread implementation for enhancing various systems, engineers are progressively integrating it into their daily work. However, due to varying levels of expertise and familiarity with AI tools among engineers, there is a need for a solution that can facilitate the expansion of the AI user community while ensuring efficient and speedy project execution. This growing demand has led to the increasing popularity of low-code solutions for AI among engineers.
Not only does it help shorten production cycles and lower project costs, low-code also bridges the gap between the latest work of data scientists and engineers on the ground. As more engineers are using AI, low-code will become a natural extension of any AI-driven work, making inter-disciplinary teams more productive and effective. But in order to fully benefit from a low-code approach, it is important engineers understand the primary applications, best practices and future trends associated with low-code for AI.
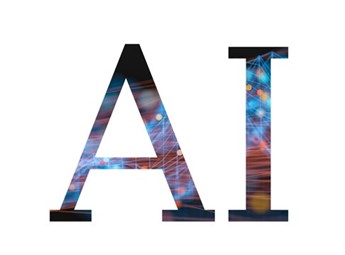
Main Uses & Advantages
Low-code has an application anywhere a data-centric approach is required, from industrial automation to radar and wireless. As such, its applications stretch throughout the entirety of the AI workflow, covering data preprocessing, modelling, simulation, testing and deployment.
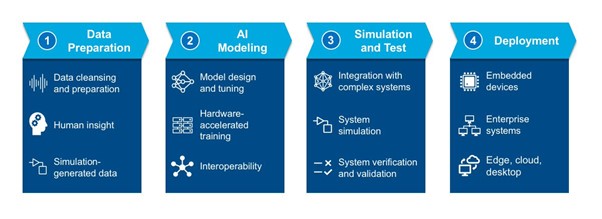
For instance, data labelling is an area that inherently lends itself to a graphical, point and click approach. As engineers work to ensure that clean training data used as input to models, point and click tools can work to remove noise from applications such as radar, wireless and signal. For example, DRASS, a marine manufacturing company, used low code image processing applications to provide clean data sets to train their autonomous surveillance systems in a challenging maritime environment. Using point and click tools within MATLAB, DRASS could standardize and manage wave motion and persistent background changes, allowing the company to complete its project within 12 months.
This interactive approach has two primary benefits. First, the ease of use inherent in solutions like point and click allows for interdisciplinary teams with different levels of coding ability to work together. As the playing field is levelled, engineers with varying levels of coding skill can collaborate to tackle the primary issue their team is facing.
This can be of particular use when monitoring multi-component systems in specific applications. Application specific low-code tools provide multifunction graphical environments for intuitive interaction with multi-component projects. For instance, apps such as Diagnostic Feature Designer provide a graphical interface for predictive maintenance scenarios, allowing engineers to evaluate potential condition indicators to understand the state of the health of machines.
Secondly, with low-code solutions engineers may be able to run variations of algorithms and quickly view tradeoffs through point and click interfaces. When multiple iterations are necessary, as is often the case working with AI, saving time on writing code across multiple tests can be vital. An example of this is Path Partner, an embedded systems company creating autonomous vehicles, who used machine learning approaches for radar-based automotive applications to cut development times. Implementing the point and click Classification Learner app to compare the efficacy of different classification algorithms in parallel, the firm was able to cut spending and development time from five months to one.
Low-code Leading the Way in Innovation
Low-code solutions also serve to help incorporate leading technology and models produced by data scientists into the graphical interfaces that engineers need.
As data scientists continue to design new models in various programming languages, these models may be harder for engineers to implement in the low code tools they prefer. For example, many research models are not built with model size constraints; however, with model compression, engineers can take the totality of that model produced by data scientists, and use point and click tools compress layers to fit onto a low cost, low powered, embedded system using a graphical environment . Through visualizing what happens when a model is compressed through apps, engineers can ensure that the model does not lose fidelity so that they may function accurately on devices of low computational power.
This is being done with the latest models in Python, yet incorporating them into MATLAB allows the transfer of the latest innovation to engineers as fast as possible.
Factors to Consider Before Implementing Low-code
While the use of low-code solutions have made it easier to manage multi-component engineering projects, it does not make incorporating AI an easy task on its own. Approaching projects with clarity of objective is still paramount. While point and click low-code tools are often used in data labelling, without knowledge of what to label, those tools cannot be effective. Similarly, low-code solutions need to be able to incorporate the technology that engineers need. If an engineer pivots from implementing machine learning algorithms to deep learning algorithms, the low-code solutions that they are using need to be able to incorporate the respective algorithms.
Another aspect to consider is time and resource allocation. As low-code solutions bring much needed speed to deadline-driven engineers, it means engineers also need to decide how to allocate the time saved in a way that benefits the overall project. For instance, with the use of low-code tools, engineers are freed up to do more sophisticated work such as driving greater accuracy through more iterative testing.
Prospects of Low-code in the Future
As more industries and companies incorporate AI into their work, implementing low-code will become a natural extension of any AI-driven work, making inter-disciplinary team work more productive and effective.
Used across applications and industries from medical to defence, low-code solutions are likely to bring an added layer of sophistication to the work engineers can produce across numerous data types. As greater involvement from an industry perspective continues, the importance of incorporating the newest machine learning and deep learning models into applications for the engineer will become more important.
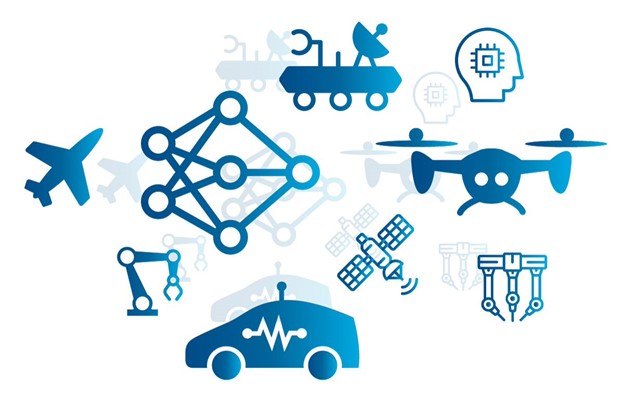